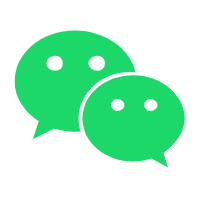
摄像头测距就是计算照片中的目标物体到相机的距离。可以使用相似三角形(triangle similarity)方法实现,或者使用更复杂但更准确的相机模型的内参来实现这个功能。
使用相似三角形计算物体到相机的距离
假设物体的宽度为 W,将其放到离相机距离为 D 的位置,然后对物体进行拍照。在照片上量出物体的像素宽度 P,于是可以得出计算相机焦距 F 的公式:
比如我在相机前 24 英寸距离(D=24 inches)的位置横着放了一张 8.5 x 11 英寸(W=11 inches)的纸,拍照后通过图像处理得出照片上纸的像素宽度 P=248 pixels。所以焦距 F 等于:
此时移动相机离物体更近或者更远,我们可以应用相似三角形得到计算物体到相机的距离的公式:
原理大概就是这样,接下来使用 OpenCV 来实现。
获取目标轮廓
# import the necessary packages
from imutils import paths
import numpy as np
import imutils
import cv2
def find_marker(image):
# convert the image to grayscale, blur it, and detect edges
gray = cv2.cvtColor(image, cv2.COLOR_BGR2GRAY)
gray = cv2.GaussianBlur(gray, (5, 5), 0)
edged = cv2.Canny(gray, 35, 125)
# find the contours in the edged image and keep the largest one;
# we'll assume that this is our piece of paper in the image
cnts = cv2.findContours(edged.copy(), cv2.RETR_LIST, cv2.CHAIN_APPROX_SIMPLE)
cnts = imutils.grab_contours(cnts)
c = max(cnts, key = cv2.contourArea)
# compute the bounding box of the of the paper region and return it
return cv2.minAreaRect(c)
定义一个 find_marker 函数,接收一个参数 iamge,用来找到要计算距离的物体。这里我们用一张 8.5 x 11 英寸的纸作为目标物体。第一个任务是在图片中找到目标物体。
下面这三行是先将图片转换为灰度图,并进行轻微模糊处理以去除高频噪声,然后进行边缘检测。
gray = cv2.cvtColor(image, cv2.COLOR_BGR2GRAY)
gray = cv2.GaussianBlur(gray, (5, 5), 0)
edged = cv2.Canny(gray, 35, 125)
做了这几步后图片看起来是这样的:
现在已经可以清晰地看到这张纸的边缘,接下来需要做的是找出这张纸的轮廓。
cnts = cv2.findContours(edged.copy(), cv2.RETR_LIST, cv2.CHAIN_APPROX_SIMPLE)
cnts = imutils.grab_contours(cnts)
c = max(cnts, key = cv2.contourArea)
用 cv2.findContours 函数找到图片中的众多轮廓,然后获取其中面积最大的轮廓,并假设这是目标物体的轮廓。
这种假设只适用于我们这个场景,在实际使用时,在图片中找出目标物体的方法与应用场景有很大关系。
我们这个场景用简单的边缘检测并找出最大的轮廓就可以了。当然为了使程序更具有鲁棒性,也可以用轮廓近似,并剔除不是四个点的轮廓(纸张是一个有四个点的矩形),然后再找出面积最大,具有四个点的轮廓。
注意: 关于这个方法,详情可以查看这篇文章,用于构建一个移动文本扫描工具。
我们也可以根据颜色特征在图片中找到目标物体,因为目标物体和背景的颜色有着很明显的不同。还可以应用关键点检测(keypoint detection),局部不变性描述子(local invariant descriptors)和关键点匹配(keypoint matching)来寻找目标。但是这些方法不在本文的讨论范围内,而且高度依赖具体场景。
我们现在得到目标物体的轮廓了,find_marker 函数最后返回的是包含轮廓 (x, y) 坐标、像素长度和像素宽度的边框,
计算距离
接下来该使用相似三角形计算目标到相机的距离。
def distance_to_camera(knownWidth, focalLength, perWidth):
# compute and return the distance from the maker to the camera
return (knownWidth * focalLength) / perWidth
distance_to_camera 函数传入目标的实际宽度,计算得到的焦距和图片上目标的像素宽度,就可以通过相似三角形公式计算目标到相机的距离了。
下面是调用 distance_to_camera 函数之前的准备:
# initialize the known distance from the camera to the object, which
# in this case is 24 inches
KNOWN_DISTANCE = 24.0
# initialize the known object width, which in this case, the piece of
# paper is 12 inches wide
KNOWN_WIDTH = 11.0
# load the furst image that contains an object that is KNOWN TO BE 2 feet
# from our camera, then find the paper marker in the image, and initialize
# the focal length
image = cv2.imread("images/2ft.jpg")
marker = find_marker(image)
focalLength = (marker[1][0] * KNOWN_DISTANCE) / KNOWN_WIDTH
首先是测量目标物体的宽度,和目标物体到相机的距离,并根据上面介绍的方法计算相机的焦距。其实这些并不是真正的摄像机标定。真正的摄像机标定包括摄像机的内参,相关知识可以可以查看这里。
使用 cv2.imread 函数从磁盘加载图片,然后通过 find_marker 函数得到图片中目标物体的坐标和长宽信息,最后根据相似三角形计算出相机的焦距。
现在有了相机的焦距,就可以计算目标物体到相机的距离了。
# loop over the images
for imagePath in sorted(paths.list_images("images")):
# load the image, find the marker in the image, then compute the
# distance to the marker from the camera
image = cv2.imread(imagePath)
marker = find_marker(image)
inches = distance_to_camera(KNOWN_WIDTH, focalLength, marker[1][0])
# draw a bounding box around the image and display it
box = cv2.cv.BoxPoints(marker) if imutils.is_cv2() else cv2.boxPoints(marker)
box = np.int0(box)
cv2.drawContours(image, [box], -1, (0, 255, 0), 2)
cv2.putText(image, "%.2fft" % (inches / 12),
(image.shape[1] - 200, image.shape[0] - 20), cv2.FONT_HERSHEY_SIMPLEX,
2.0, (0, 255, 0), 3)
cv2.imshow("image", image)
cv2.waitKey(0)
使用 for 循环遍历每个图片,计算每张图片中目标对象到相机的距离。在结果中,我们根据得到的轮廓信息将方框画了出来,并显示出了距离。下面是得到的几个结果图:
总结
通过这篇文章,我们学会了使用相似三角形计算图片中一个已知物体到相机的距离。
需要先测量出目标物体的实际宽度和目标物体到相机的距离,然后使用图像处理的方法自动计算图片中目标物体的像素宽度,并使用相似三角形计算出相机的焦距。
根据相机的焦距就可以计算图片中的目标物体到相机的距离。
申明:感谢原创作者的辛勤付出。本号转载的文章均会在文中注明,若遇到版权问题请联系我们处理。
----与智者为伍为创新赋能----

联系邮箱:uestcwxd@126.com
QQ:493826566
暂无评论哦,快来评论一下吧!
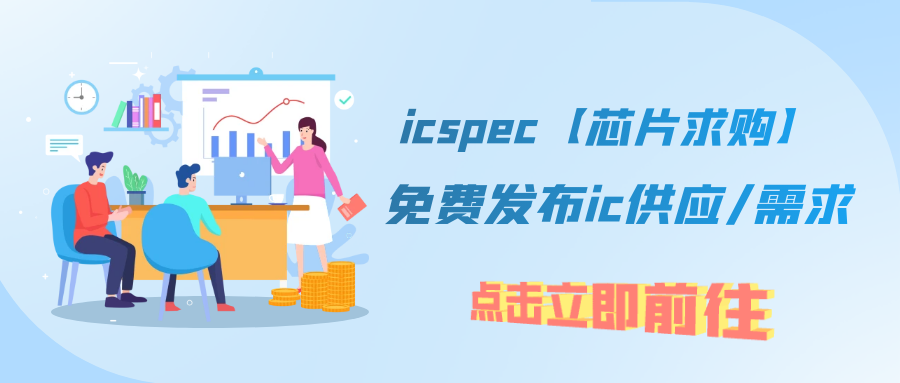
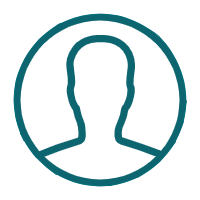